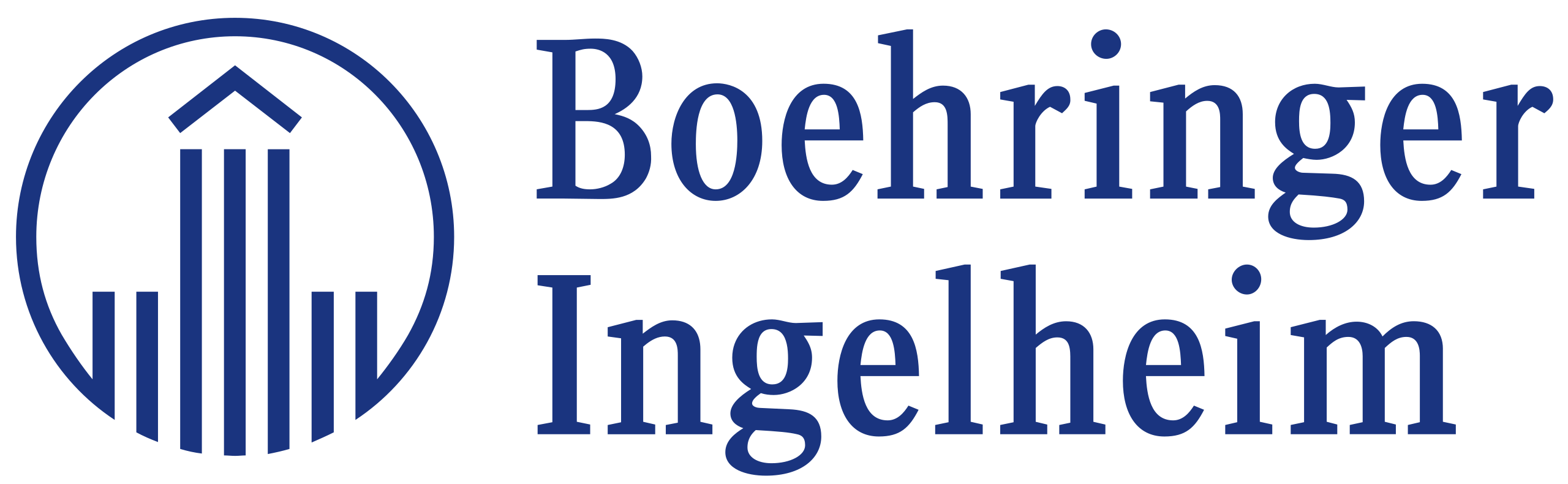
Generative AI as a personal agent for knowledge management processes
Unleash the power of Artificial Intelligence and generative models in the pharmaceutical industry together with Boehringer Ingelheim by developing personalized AI agents that support health workers in knowledge management, research & content creation.
Shape the future of human-machine interaction and master demanding challenges in the pharmaceutical industry together.
#KnowledgeManagement #PersonalAIagent #GenerativeAI
✅ Challenge completed 🏁 Winner Congratulations to Curiousity AI (1st place) & Arkangel AI (2nd place) for winning the challenge!🏆 Rewards EUR 3,000 prize pool + versatile collaboration models + hiring opportunities🌱 Innovate 2030 All SDGs This challenge is part of the INNOVATE2030 program
Brief
The Challenge
Submission
Rewards
Opportunity Areas
FAQ
Here is a brief overview of the content and what to expect when joining the challenge. Further, more detailed information can be found in the following tabs.
© 2018-2023 ekipa GmbH. All rights reserved.