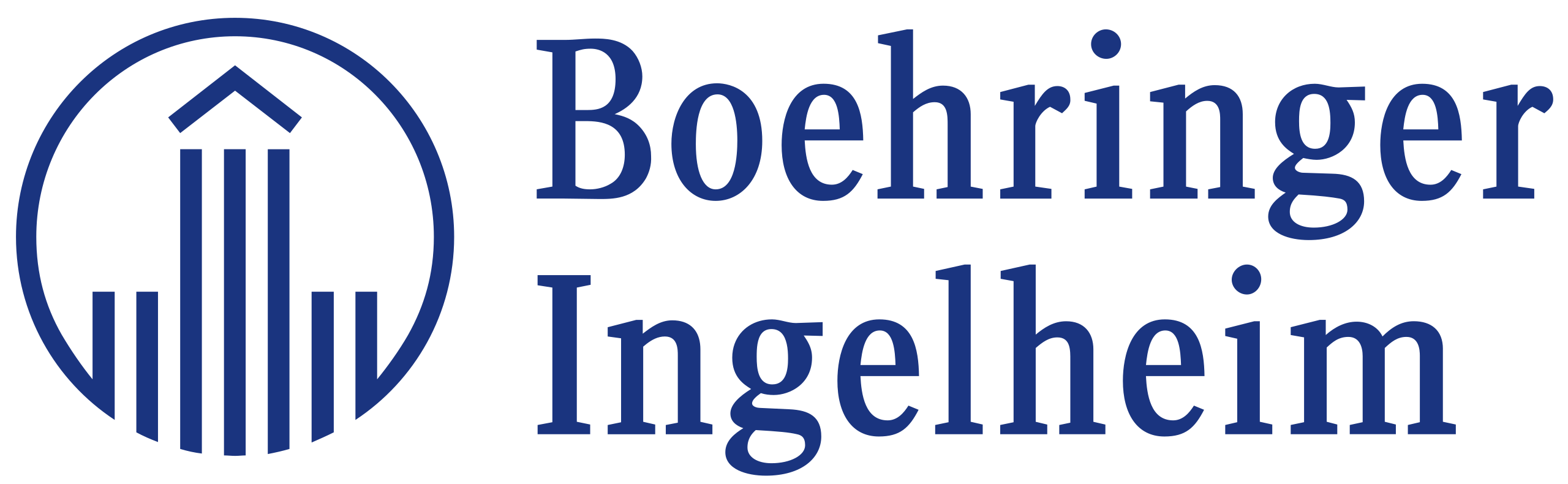
Generative AI as a personal agent for knowledge management processes
Unleash the power of Artificial Intelligence and generative models in the pharmaceutical industry together with Boehringer Ingelheim by developing personalized AI agents that support health workers in knowledge management, research & content creation.
#KnowledgeManagement #PersonalAIagent #GenerativeAI
✅ Challenge completed 🏁 Winner Congratulations to Curiousity AI (1st place) & Arkangel AI (2nd place) for winning the challenge!🏆 Rewards Funded Co-Creation + versatile collaboration models for implementation📃 Venture clienting BI is searching for a long-term partner to implement the solution
To develop a comprehensive solution for effective knowledge management, it is advised to take a deeper look at these two areas: Human Machine Interface & Technology Development
HUMAN MACHINE INTERFACE
The Human Machine Interface is a critical aspect of the knowledge platform, as it determines how users interact with the system. It encompasses the design and implementation of user interfaces, navigation structures, and interaction mechanisms. The HMI should be intuitive, user-friendly, and tailored to the specific needs of the pharmaceutical industry.
Therefore, in this step the interaction model and the user interface should be demonstrated.
TECHNOLOGY DEVELOPMENT
Technology development refers to the process of building the AI model that powers the knowledge platform. This involves various stages, including data acquisition, preprocessing, model training, and validation. The elaboration should highlight and explain the following functions in particular:
1. Text analysis
Text analysis is a fundamental step in the knowledge management process. It involves using natural language processing techniques to understand and extract meaningful information from text documents. By applying text analysis, key concepts, entities, relationships, and sentiments present in the text can be identified, organizing and categorizing the information, making it easier to retrieve and utilize for further processing.
2. Automatic summarization
Automatic summarization aims to condense large volumes of text into shorter summaries while preserving the essential information and maintaining coherence. By leveraging techniques like extractive or abstractive summarization, the system identifies the most important sentences or phrases and generates a concise summary.
3. New article & topic generation
New article generation involves using generative models to create new articles based on existing knowledge. By utilizing techniques like language models or transformer architectures, the system can generate coherent and contextually relevant articles. This step goes beyond summarization and allows for the generation of original content by leveraging the knowledge and insights extracted from various sources. In addition, it is also relevant that from the given information and topics, based on previous articles and knowledge from the sources, the model can also generate new topic suggestions, that are not covered yet.
4. Validation
Validation is a further key aspect. This involves the development of appropriate evaluation metrics to assess the quality of the generated articles. These metrics may include measures such as coherence, factual accuracy, readability, relevance to the input data, and adherence to specific guidelines or criteria.
© 2018- 2024 ekipa GmbH. All rights reserved.