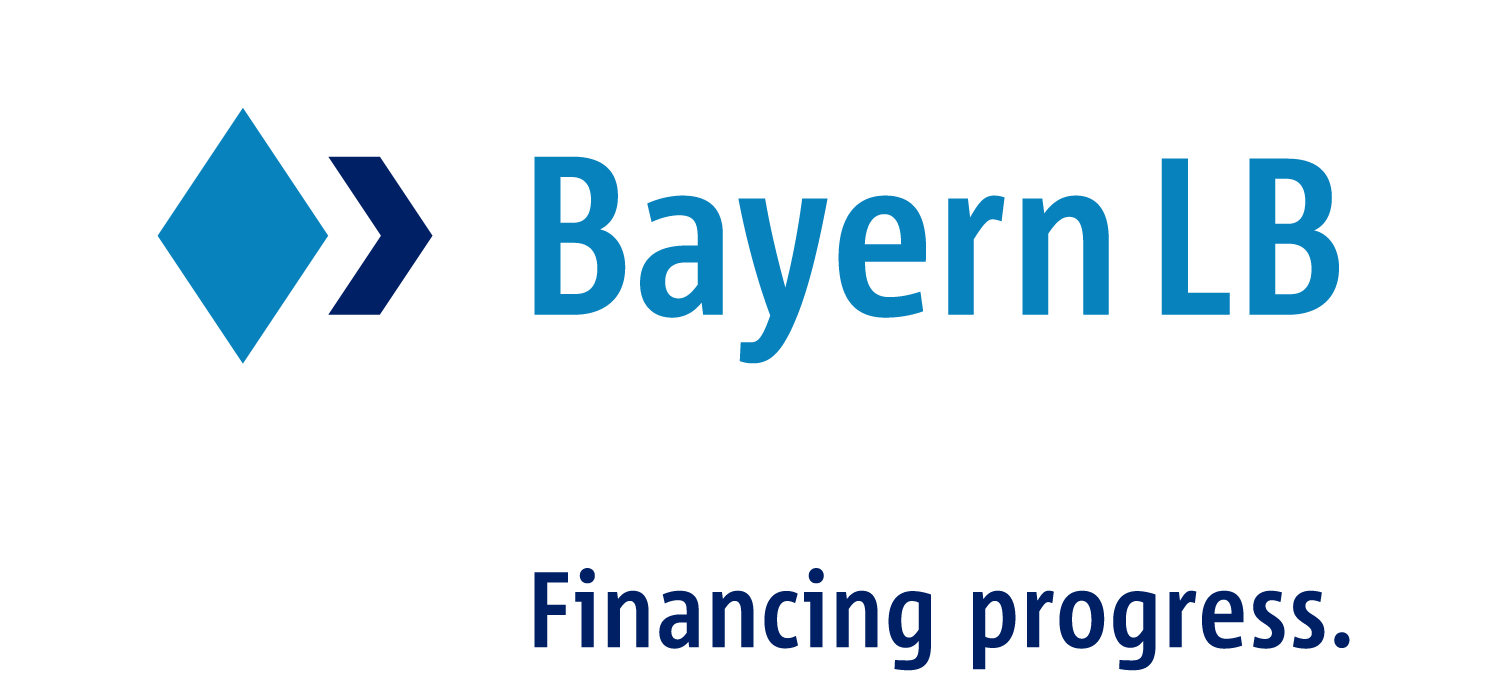
Use AI to revolutionize banks' risk management and decision-making
Join our challenge to revolutionize banks' risk management! We are seeking innovative solutions that integrate Large Language Models with traditional quantitative analytics to predict financial risks with greater accuracy. Unleash your creativity and redefine banking risk assessment!
Who can participate?
Start-ups, (technology) companies, researchers, labs, newly founded tech teams and all other AI innovators
#AIRiskPrediction #FinancialAnalytics #AIinBanking
🏆 Rewards Funded co-creation, strategic partnerships & long-term integration🕑 Deadline Jul 9, 2024, 9:59:00 PM🌎 Scope Global
❓Questions Feel free to join our Q&A Calls
👥 Looking for a team? Join our Team Matching Channel
In this tab, you can find all the relevant information for your submission. The following contents must be submitted for participation
How to submit
- Submission template (mandatory)
A standard template is available. The template will help you structure your solutions and approaches and provide answers to all relevant questions and frameworks. Download the Submission Template here - Optional Content
You can try to stand out and also supplement your submission with prototypes, image and video materials, or other concepts or visual elements - simply submit these files additionally via our platform.
Key Guidelines and Specifications:
Develop the concept/prototype (if applicable) according to the following criteria:
- Data Synergy: Explore creative approaches to integrate traditional financial data with qualitative insights from publicly available sources, such as annual reports, news articles, internal intranet entries, trends, and Google News, thereby enhancing the depth of risk analysis.
- Content Quality: Ensure the content and data used by the tool are of high quality and relevant to the financial sector.
- Effective Data Use: The tool should effectively use integrated data sources, demonstrating the ability to handle and interpret large and diverse datasets.
- Predictive Power and Explainability: Aim to build a tool that not only analyzes but also predicts future events and financial developments, offering well-founded rationales for its conclusions. Prioritize the clarity and justification of the tool's predictions, enhancing its trustworthiness, reliability and explainability.
- Usability: Design the concept/tool to be user-friendly for risk managers, research teams, and sales departments.
- Regulatory Alignment: Ensure the solution aligns with EU data security and privacy regulations. Cyber and data security is a number one priority for banks.
- Comprehensive concept: Develop a well-rounded, detailed concept during the qualification phase that transcends basic theoretical frameworks and is primed for further refinement in a specific application environment. Submissions must showcase a broad understanding of the context, offer feasible and insightful solutions, and present a clear, innovative approach, illustrating how the concept could be practically applied and scaled in real-world scenarios. The concept should provide a solid foundation for future development into a functional prototype or a full-scale solution, demonstrating its potential impact and viability in a banking environment.
Optional:
The following aspects can be considered but are not mandatory:
- Functional Prototype: Create a first tangible, working prototype during the qualification phase that goes beyond theoretical ideas and can be further developed in a banking environment. Submissions should demonstrate practical applicability, reasonable predictions, and an innovative, clear concept, demonstrating the usability of the tool in real-world banking scenarios.
- Use of Microsoft Azure: Using Microsoft’s Azure AI Services is a plus.
- Industry or Asset Class Focus: Participants may choose to tailor the solution to a specific industry or asset class to reduce complexity. This focus could enhance the tool’s effectiveness in particular sectors, providing deeper insights tailored to unique market dynamics or risk factors. Retail business should be excluded from the analysis. The following industries and/or asset classes are relevant to BayernLB:
BayernLB Factbook
• Real Estate
• Renewable Energy
• Mobility: Automotive & Logistics, Rail & Bus, Aviation & Space
• Technology, IT & Telecom
• Grid Operators & Utilities
• Manufacturing & Engineering
• Construction & Infrastructure
• Materials
- Focus on Europe and the USA: We do not expect the solution to cover the whole world in detail. Focus on Europe and the USA is sufficient.
- Predictions based on Changes of Probabilities: Given the high complexity and difficulty of predicting future events, participants could work with changes of probabilities. Instead of making explicit predictions about future events the solution could constantly assess probabilities. Significant changes in these probabilities could give valuable insights on the likelihood of occurrences of future events irrespective of the absolute level of the probability.
- One Set of Predictions per Day: We do not expect the tool to produce predictions on a continuous/real-time basis. One prediction per day is enough. However, the output should be up to date without huge time delays.
- Backtesting: A concept for backtesting and/ or evaluating the model’s accuracy is a plus.
© 2018- 2024 ekipa GmbH. All rights reserved.